STELA – Study Success Utilising Learning Analytics
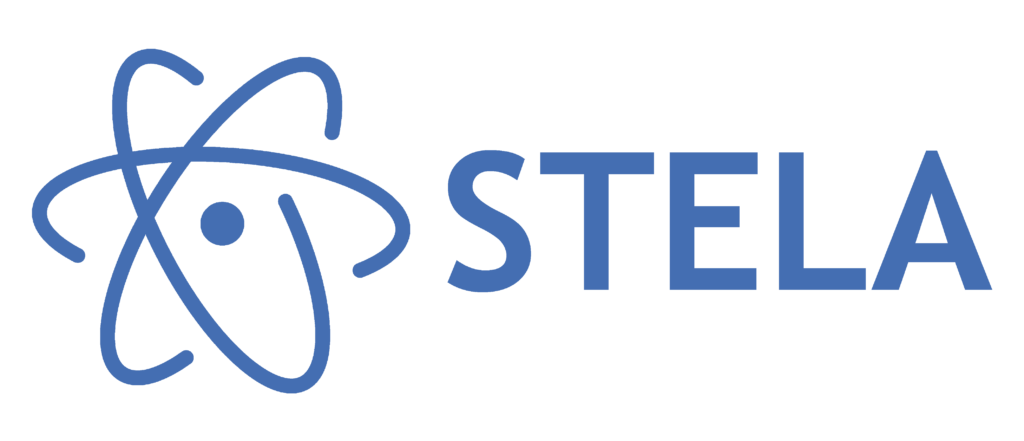
The aim of the project “Utilizing Learning Analytics for Study Success” is to conduct a systematic review and construct a set of policies for higher education institutions to adopt LA capabilities into their existing learning environments. Precisely, the goals of our project are 1) first to build a systematic review of empirical evidence demonstrating how learning analytics have been successful in facilitating student success in continuation and completion of their university courses both nationally and internationally (focus of this paper), and forming the basis for aim no. 2) to make policy recommendations for the higher education sector in order to accept and implement such systems within institutions.
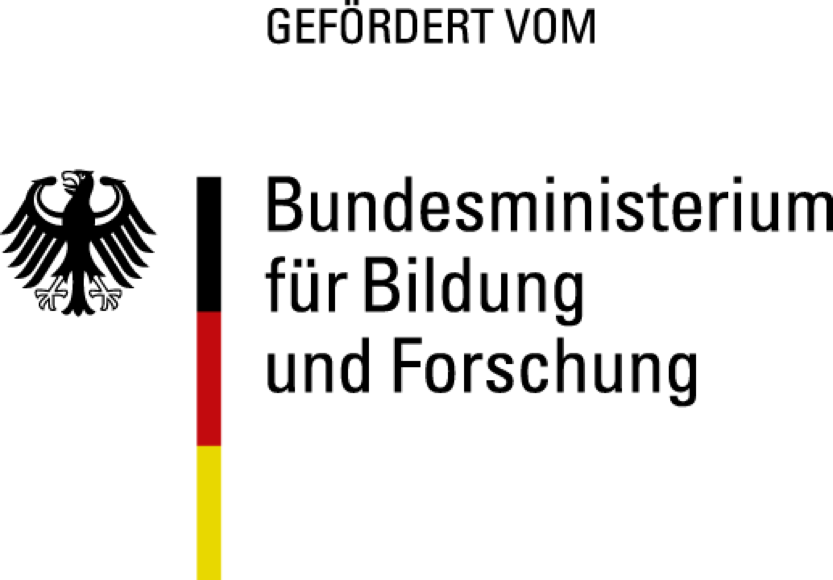
Selected publications related to the project
Ifenthaler, D., Yau, J. Y.-K., & Mah, D.-K. (Eds.). (2019). Utilizing learning analytics to support study success. New York, NY: Springer.
Ifenthaler, D., Mah, D.-K., & Yau, J. Y.-K. (2019). Utilising learning analytics for study success. Reflections on current empirical findings. In D. Ifenthaler, J. Y.-K. Yau, & D.-K. Mah (Eds.), Utilizing learning analytics to support study success (pp. 27–36). New York, NY: Springer.
Ifenthaler, D., & Yau, J. (2019). Higher education stakeholders’ views on learning analytics policy recommendations for supporting study success. International Journal of Learning Analytics and Artificial Intelligence for Education, 1(1), 28–42. doi:10.3991/ijai.v1i1.10978
Mah, D.-K., Yau, J. Y.-K., & Ifenthaler, D. (2019). Future directions on learning analytics to enhance study success. In D. Ifenthaler, J. Y.-K. Yau, & D.-K. Mah (Eds.), Utilizing learning analytics to support study success (pp. 313–321). New York, NY: Springer.
LeAP – Learning Analytics Profile

The field of learning analytics (LA) is generating growing interest in data and computer science as well as educational science, hence, becoming an important aspect of modern e-learning environments. However, implementing learning analytics into existing legacy systems in learning environments with high data privacy concerns is quite a challenge. This research shows how a learning analytics application has been implemented into such an existing university environment by adding a plugin to the local digital learning environment which injects user centric information to specific objects within students’ and teachers’ learning environment.
Selected publications related to the project
Ifenthaler, D., & Schumacher, C. (2019). Releasing personal information within learning analytics systems. In D. G. Sampson, J. M. Spector, D. Ifenthaler, P. Isaias, & S. Sergis (Eds.), Learning technologies for transforming teaching, learning and assessment at large scale (pp. 3–18). New York, NY: Springer.
Klasen, D., & Ifenthaler, D. (2019). Implementing learning analytics into existing higher education legacy systems. In D. Ifenthaler, J. Y.-K. Yau, & D.-K. Mah (Eds.), Utilizing learning analytics to support study success (pp. 61–72). New York, NY: Springer.
Schumacher, C., Klasen, D., & Ifenthaler, D. (2019). Implementation of a learning analytics system in a productive higher education environment In M. S. Khine (Ed.), Emerging trends in learning analytics (pp. 177–199). Leiden‚ NL: Brill.
Schumacher, C., & Ifenthaler, D. (2018). Features students really expect from learning analytics. Computers in Human Behavior, 78, 397–407. doi:10.1016/j.chb.2017.06.030
Schumacher, C., & Ifenthaler, D. (2018). The importance of students’ motivational dispositions for designing learning analytics. Journal of Computing in Higher Education, 30(3), 599–619. doi:10.1007/s12528-018-9188-y
Ifenthaler, D. (2017). Are higher education institutions prepared for learning analytics? TechTrends, 61(4), 366–371. doi:10.1007/s11528-016-0154-0
Ifenthaler, D., & Schumacher, C. (2016). Student perceptions of privacy principles for learning analytics. Educational Technology Research and Development, 64(5), 923–938. doi:10.1007/s11423-016-9477-y
Educational Data Literacy
Educational Data Literacy (EDL) is the ethically responsible collection, management, analysis, comprehension, interpretation, and application of data from educational contexts.
With the growing availability of educational data stemming from the digital learning environments and related learning and teaching processes as well as their contexts, instructional designers, e-Trainers, and teachers (among other stakeholders) gain significant information for enhancing on-demand personalised educational support of individual learners as well as reflective course (re)design for achieving more authentic training, learning and assessment experiences integrated into real work oriented tasks. However, data-driven competencies for instructional designers, e-Trainers, or teachers are not yet comprehensively addressed by existing competence frameworks and professional development programs.
This research project on Educational Data Literacy develops and validates a framework and provides professional learning opportunities for stakeholders.
Related project
Learn2Analyze (L2A) is an Academia-Industry Knowledge Alliance for enhancing Online Training Professionals’ (Instructional Designers and e-Trainers) Competences in Educational Data Analytics, co-funded by the European Commission through the Erasmus+ Program of the European Union (Cooperation for innovation and the exchange of good practices – Knowledge Alliances, Agreement n. 2017-2733 / 001-001, Project No 588067-EPP-1-2017-1-EL-EPPKA2-KA) for the period 2018-2020.
Learn2Analyze Educational Data Literacy Competence Profile (L2A-EDL-CP)
The Learn2Analyze project has developed a comprehensive proposal for an Educational Data Literacy Competence Framework to enhance existing competence frameworks for instructional designers and e-trainers of online courses with new Educational Data Literacy competences.
The Learn2Analyze Educational Data Literacy Competence Framework comprises of 6 competence dimensions and 17 competence statements, as captured below.
L2A-EDL-CP
Dimensions
L2A-EDL-CP
Statements
1. Data Collection
1.1 Know – understand – be able to obtain, access and gather the appropriate data and/or data sources
1.2 Know – understand – be able to apply data limitations and quality measures (e.g., validity, reliability, biases in the data, difficulty in collection, accuracy, completeness)
2. Data Management
2.1 Know – understand – be able to apply data processing and handling methods (i.e., methods for cleaning and changing data to make it more organized – e.g., duplication, data structuring)
2.2 Know – understand – be able to apply data description (i.e., metadata)
2.3 Know – understand – be able to apply data curation processes (i.e., to ensure that data is reliably retrievable for future reuse, and to determine what data is worth saving and for how long)
2.4 Know – understand – be able to apply the technologies to preserve data (i.e., store, persist, maintain, backup data), e.g., storage mediums/services, tools, mechanisms
3. Data Analysis
3.1 Know – understand – be able to apply data analysis and modeling methods (e.g. application of descriptive statistics, exploratory data analysis, data mining).
3.2 Know – understand – be able to apply data presentation methods (e.g., pictorial visualization of the data by using graphs, charts, maps and other data forms like textual or tabular representations)
4. Data Comprehension & Interpretation
4.1 Know – understand – be able to interpret data properties (e.g., measurement error, outliers, discrepancies within data, key take-away points, data dependencies)
4.2 Know – understand – be able to interpret statistics commonly used with educational data (e.g., randomness, central tendencies, mean, standard deviation, significance)
4.3 Know – understand – be able to interpret insights from data analysis (e.g., explanations of patterns, identification of hypotheses, connection of multiple observations, underlying trends)
4.4 Be able to elicit potential implications/links of the data analysis insights to instruction
5. Data Application
5.1 Know – understand – be able to use data analysis results to make decisions to revise instruction
5.2 Be able to evaluate the data-driven revision of instruction
6. Data Ethics
6.1 Know – understand – be able to use the informed consent
6.2 Know – understand – be able to protect individuals’ data privacy, confidentiality, integrity and security
6.3 Know – understand – be able to apply authorship, ownership, data access (governance), re-negotiation and data-sharing